Expanded Cross-entropy Loss for Convolutional Neural Networks
The loss function extremely affects the performance of the model. How to define the loss function determines whether a learning is successful or not. The Cross-entropy loss is commonly used in the model for classification. It calculates the loss value only through information about a correctly predicted probability. This paper proposes the Expanded Cross-entropy loss function that complements the Cross-entropy loss function. The Expanded Cross-entropy loss calculates the weighted sum of the Cross-entropy loss and an additional term containing information about incorrectly predicted probabilities.
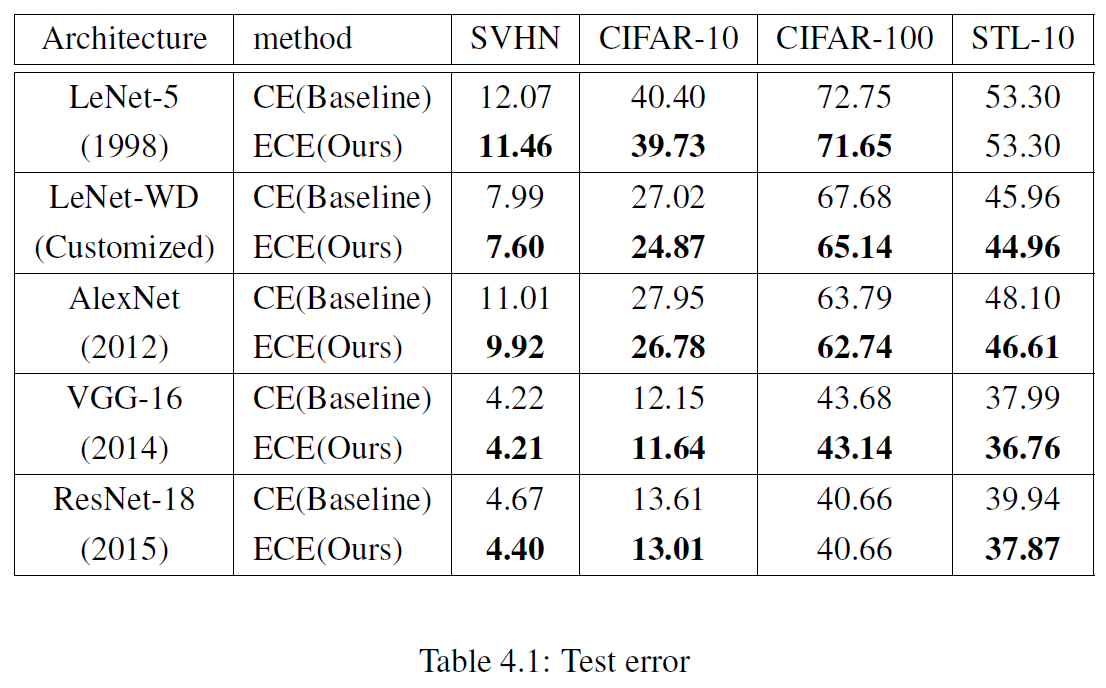
For SVHN, CIFAR-10, CIFAR-100, and STL-10 dataset, we evaluated the accuracy of convolutional neural networks with various architectures. In most cases, assuming all the other conditions are in same state, the Expanded Cross-entropy loss function has been found to increase the accuracy of classification compared to the Cross-entropy loss function.
SVHN
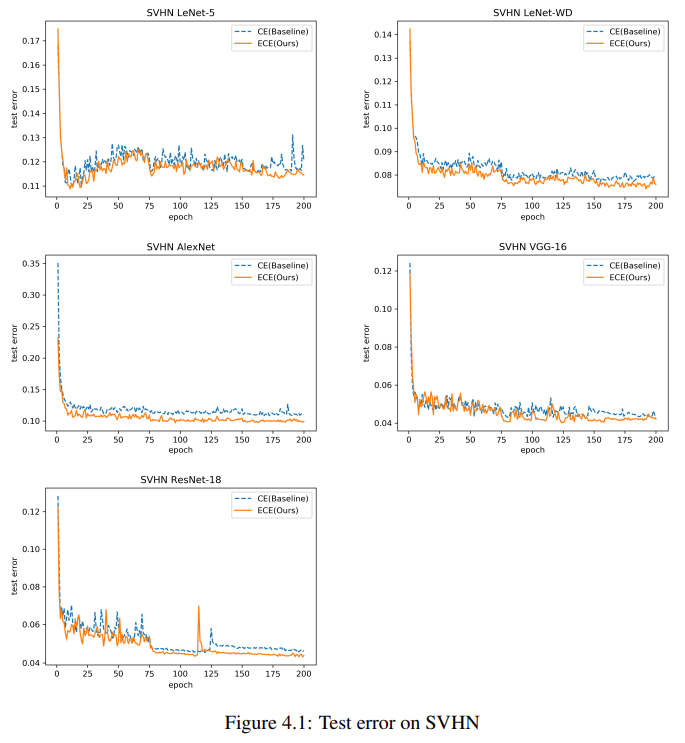
CIFAR-10
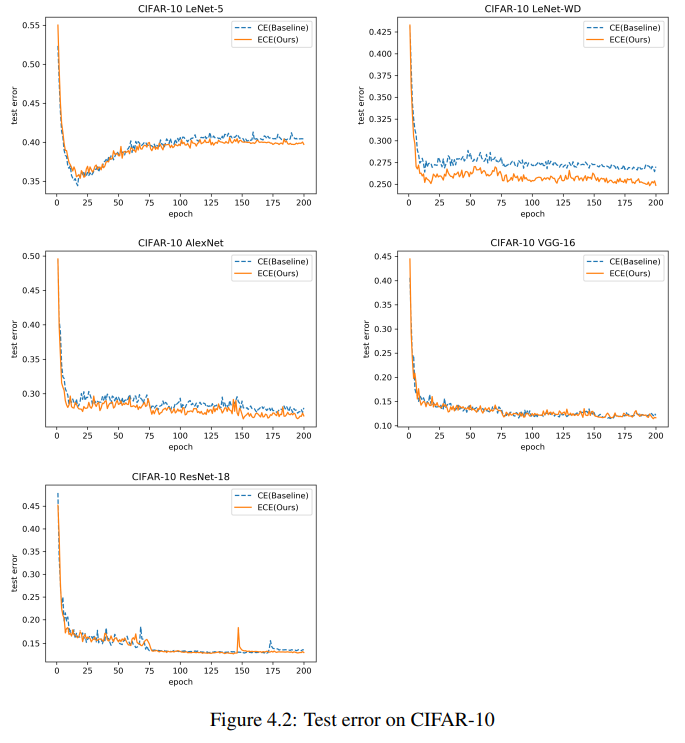
CIFAR-100
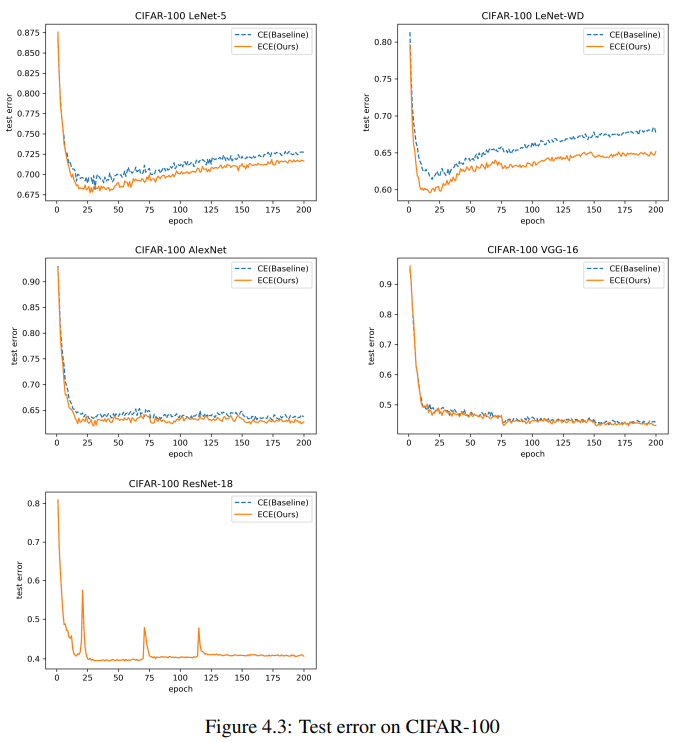
STL-10
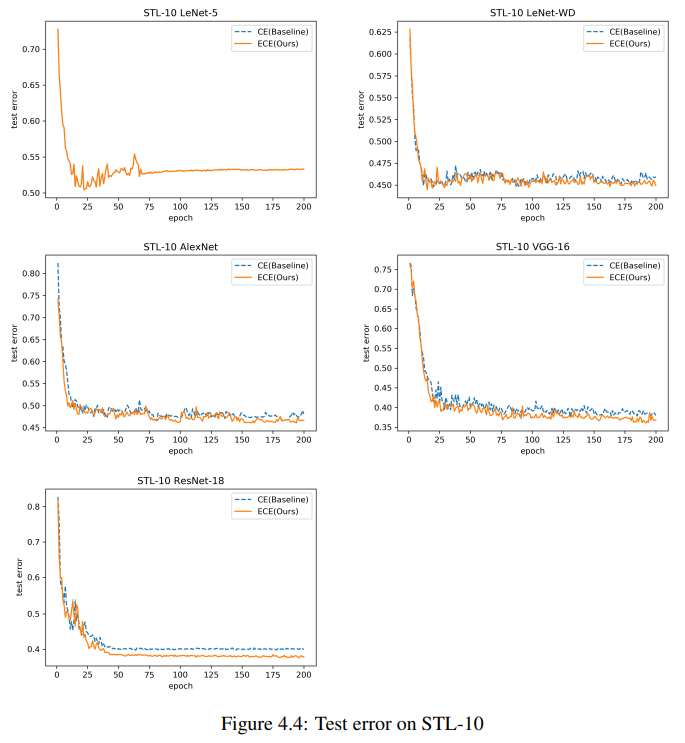